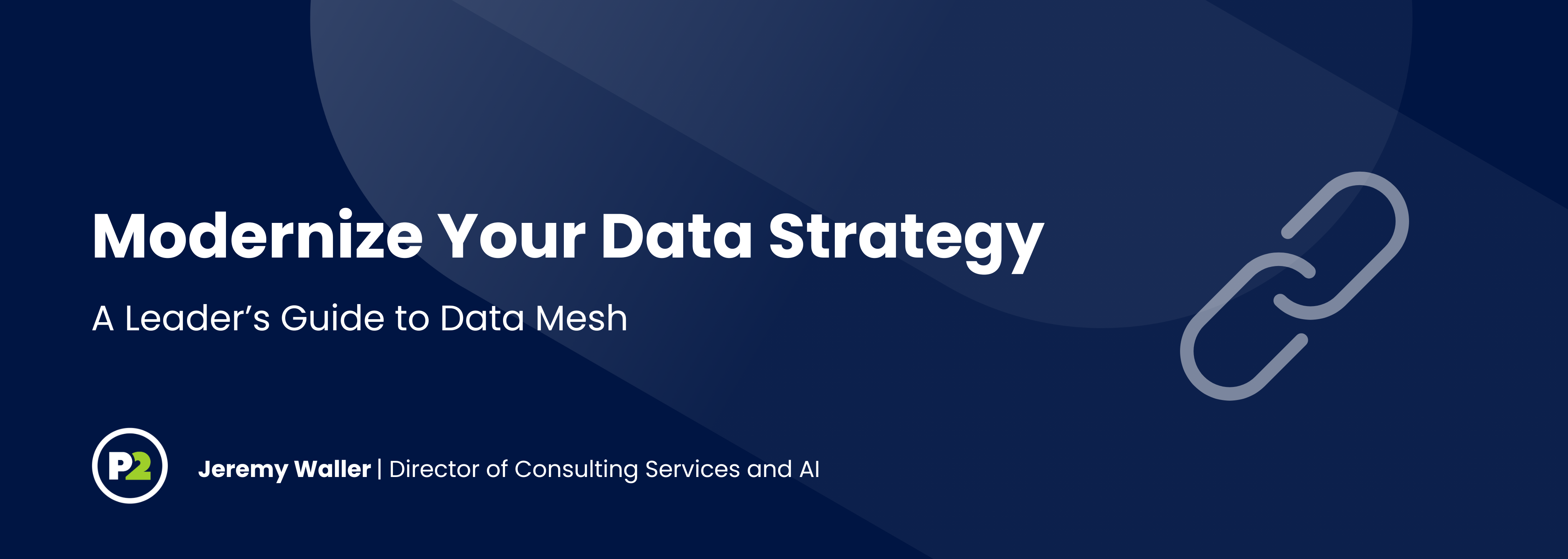
Modernize Your Data Strategy: A Leader’s Guide to Data Mesh
Today’s organizations face an unprecedented explosion in data volume, variety, and velocity. The promise of data-driven decision making often remains out of reach as traditional centralized data architectures show their limitations. Key challenges include:
- Data teams becoming organizational bottlenecks
- Business units waiting weeks for simple changes
- Valuable insights trapped in departmental silos
- Rising costs of maintaining centralized data infrastructure
Imagine this scenario: The marketing team needs urgent insights about customer behavior during a major promotion. The data exists, scattered across e-commerce platforms, CRM systems, and social media analytics. However, the central data team is already backlogged with quarter-end requests from finance and sales. By the time marketing receives their analysis, the promotional opportunity has passed, leaving revenue on the table and competitors free to capture market share.
This plays out daily across industries, and it’s more than just an inconvenience—it’s a strategic liability in today’s fast-paced business environment.
The Promise of Data Mesh
A data mesh represents a fundamental shift in how we think about and manage enterprise data. Rather than treating data as a byproduct that needs to be centrally collected and processed, it views data as a first-class product that should be owned and managed by those closest to its creation and use.
The core principles that make a data mesh transformative:
- Domain ownership of data: Moving responsibility to the teams who understand the data best, enabling faster decisions and ensuring data serves real business needs
- Data treated as a product: Approaching data with the same rigor as commercial products, complete with clear documentation, quality standards, and expected outcomes
- Self-service infrastructure: Providing teams with the tools and platforms they need to store, process, and share data independently, while maintaining security standards
- Federated governance: Balancing organizational consistency with domain autonomy through shared standards for data quality, security, and compliance that can be implemented flexibly across different business contexts while maintaining enterprise-wide security controls
Real-World Impact: Beyond the Private Sector
While most organizations implement a data mesh to solve commercial challenges, its principles can transform public sector organizations as well. Consider how a state-level environmental protection agency might tackle the challenge of monitoring and managing water quality across hundreds of locations and multiple watersheds.
In this scenario, each regional office may maintain its own monitoring systems and reporting methods, making it difficult to get a comprehensive view of water quality trends and potential issues. Any centralized office would struggle to standardize and integrate data from dozens of sources, leading to delays in identifying and responding to environmental concerns.
By implementing a data mesh approach, the agency could empower each regional office to create standardized data products while maintaining local control over their monitoring systems. This could dramatically improve response times, enhance data quality through local ownership, and give decision-makers access to reliable, real-time information. Most importantly, the improved data flow could enable better resource allocation and more proactive environmental protection measures.
The Implementation Journey
While technology enables a data mesh, the true challenges—and opportunities—lie in people and processes. Successfully implementing a data mesh requires organizational transformation led by leaders who can articulate a compelling vision and sustain momentum through inevitable obstacles. A data mesh isn’t just a technical architecture—it’s a fundamental shift in how an organization thinks about and works with data.
With that in mind, organizations typically progress through three distinct phases on their path to a data mesh, each requiring different types of leadership and focus.
Foundation: Setting the Stage
The first three to six months focus on building the groundwork for success. During this phase, resistance to distributed ownership often emerges as teams grapple with new responsibilities. Success requires leaders who can paint a clear picture of the future state while acknowledging the challenges of the journey ahead. Most importantly, leaders must demonstrate their commitment through actions, not just words—whether that’s allocating resources, removing obstacles, or actively participating in the transformation themselves.
Pilot Implementation: Learning by Doing
The next six to nine months focus on developing and publishing the first true data products. This phase tests not just technical frameworks but organizational readiness for change. Teams must adopt new mindsets, thinking like product managers rather than data custodians. They need to consider their data consumers’ needs, establish service-level agreements, and create clear documentation. A key milestone in this phase is establishing a self-service data marketplace where these products are published, discovered, and consumed by other teams. This marketplace becomes the foundation for cross-domain data sharing, enabling teams to independently discover and access data products without going through central bottlenecks.
While technical challenges will arise, the bigger hurdles often involve changing ingrained habits and ways of working. Teams must learn to think both as producers and consumers of data products, understanding how to effectively publish their own data while learning to discover and utilize data products from other domains. Success stories from the pilot teams become crucial tools for building broader organizational buy-in.
Scale and Optimize: Building Momentum
In the scale and optimize phase, lasting nine to eighteen months, organizations begin to realize the true potential of a data mesh. As more domains come online, the focus shifts to fostering a collaborative data culture. Teams begin to discover novel ways to combine and analyze data that weren’t possible under the old centralized model. However, maintaining momentum during this phase requires continued leadership attention. It’s easy for teams to slip back into old habits when faced with deadlines or pressure.
Leading the Transformation
Throughout all phases, success depends on leaders who understand that this initiative is more about organizational change than technology. They must commit to the transformation while giving teams the space and support to adapt to new ways of working. By focusing on quick wins and sharing success stories, leaders can build momentum and demonstrate the value of this new approach to data management.
Measuring Success and Navigating Challenges
Success in a data mesh transformation manifests in both operational improvements and business outcomes. Early wins often come from operational metrics: faster delivery of data products, reduced time to integrate new data sources, and fewer bottlenecks in data access. But the true value emerges in business outcomes: faster time-to-market for data-driven initiatives, increased cross-domain innovation, and new insights that drive business decisions.
Leading organizations track metrics that matter to their specific context:
- For customer-facing teams: faster response to market changes, more personalized customer experiences, reduced time to launch new offerings
- For operations teams: improved forecast accuracy, reduced waste, better resource allocation
- For product teams: shorter development cycles, more effective A/B testing, data-driven feature prioritization
- For finance teams: more accurate reporting, better risk assessment, improved fraud detection
- For public sector teams: improved service delivery, better policy outcomes, increased transparency in decision-making
Common Pitfalls and How to Avoid Them
While every organization’s journey is unique, certain challenges appear consistently:
Over-decentralization occurs when domains operate too independently, creating new silos instead of breaking them down. The antidote isn’t more central control, but rather clear standards and strong cross-domain collaboration practices. Successful organizations establish common data formats, quality standards, and sharing protocols while allowing domains flexibility in how they meet these standards.
Under-investment in shared infrastructure can cripple scalability just as teams begin to see the benefits of the data mesh. Organizations need more than just storage and compute resources—they need robust tools for data discovery, quality monitoring, and cross-domain collaboration. Smart organizations treat this infrastructure as a product itself, continuously improving it based on domain team feedback.
Low marketplace adoption occurs when organizations build data products without understanding consumer needs. Teams might publish data to the marketplace, but without clear documentation, reliable SLAs, or demonstrated value, no one will use it. Successful organizations treat their data marketplace like a product itself, actively monitoring usage patterns, gathering consumer feedback, and ensuring data products maintain promised freshness and quality levels.
The “if you build it, they will come” fallacy happens when organizations focus on technical implementation without adequate attention to adoption. Success requires actively identifying valuable use cases where teams can benefit from being both producers and consumers in the data marketplace. Organizations should track not just how many data products are published, but how many are actively used, whether they meet their SLAs, and what value they deliver to consumers.
Analysis paralysis can set in when teams overthink governance and standards. While these are crucial, the best approach is to start with minimal viable governance and evolve based on actual needs and challenges. This allows organizations to learn from experience rather than trying to anticipate every possible scenario.
Balancing Freedom and Control
Perhaps the biggest challenge is striking the right balance between domain autonomy and enterprise-wide consistency. Too much central control stifles innovation and agility—the very problems a data mesh aims to solve. Too little coordination leads to chaos and incompatibility.
Successful organizations find this balance by:
- Establishing clear principles rather than rigid rules
- Creating feedback loops between domain teams and central platform teams
- Focusing governance on outcomes rather than procedures
- Building communities of practice to share learning across domains
- Measuring and incentivizing both domain success and cross-domain collaboration
Moving Forward
Starting a data mesh transformation requires careful preparation, but don’t let the scope of change become paralyzing. Begin with an honest assessment of your organization’s readiness: Where are your current data bottlenecks? Which domains show the most promise for early success? What capabilities need strengthening?
The most successful organizations approach this journey with both ambition and pragmatism. They choose pilot domains that can demonstrate tangible value quickly. They invest in building the right capabilities—both human and technical. Most importantly, they recognize that perfection isn’t the goal; continuous learning and adaptation are.
The pressure to become more data-driven isn’t going away. Traditional approaches to data management are showing their limitations, especially as organizations grow in complexity and data needs evolve. A data mesh offers a path forward that balances enterprise-wide consistency with domain-level agility.
Ready to explore how you can transform your organization’s relationship with data? Contact us and let’s discuss your specific challenges and opportunities.